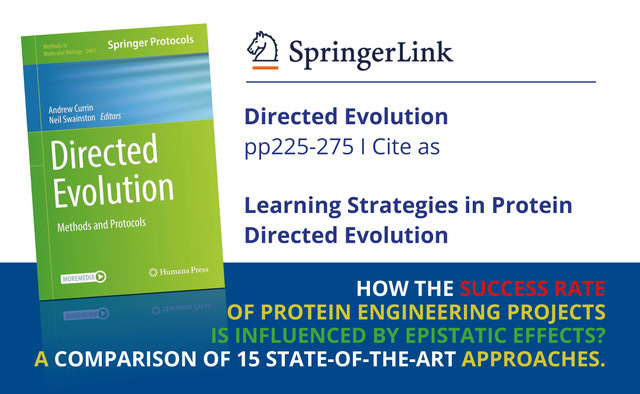
The recent development of structure prediction deep learning (DL) tools such as Alphafold2 (DeepMind), ESMfold (Meta) or ProteinMPNN (David Baker’s team) has revolutionized this area. Nevertheless, these DL tools are not suitable for predicting how individual amino acid changes alter protein function: they can’t predict epistaticeffects.
After protein folding powered by DeepMind and Meta the next challenge is to accurately predict epistasis ie. the impact of non-linear interactions of mutations within the protein sequence on the function.
Our recent review of Machine Learning (ML) and Deep Learning (DL) strategies examines how epistatic effects influence the success rate of protein engineering projects by comparing 15 state-of-the-art approaches (see Table 4: https://link.springer.com/protocol/10.1007/978-1-0716-2152-3_15/tables/4) and provides a general workflow for non-experts when using such learning strategies.
Read more: https://link.springer.com/protocol/10.1007/978-1-0716-2152-3_15#Abs1
For more information:
PEACCEL
Making the world disease free
Contact: AI-team@peaccel.com
http://www.peaccel.com/