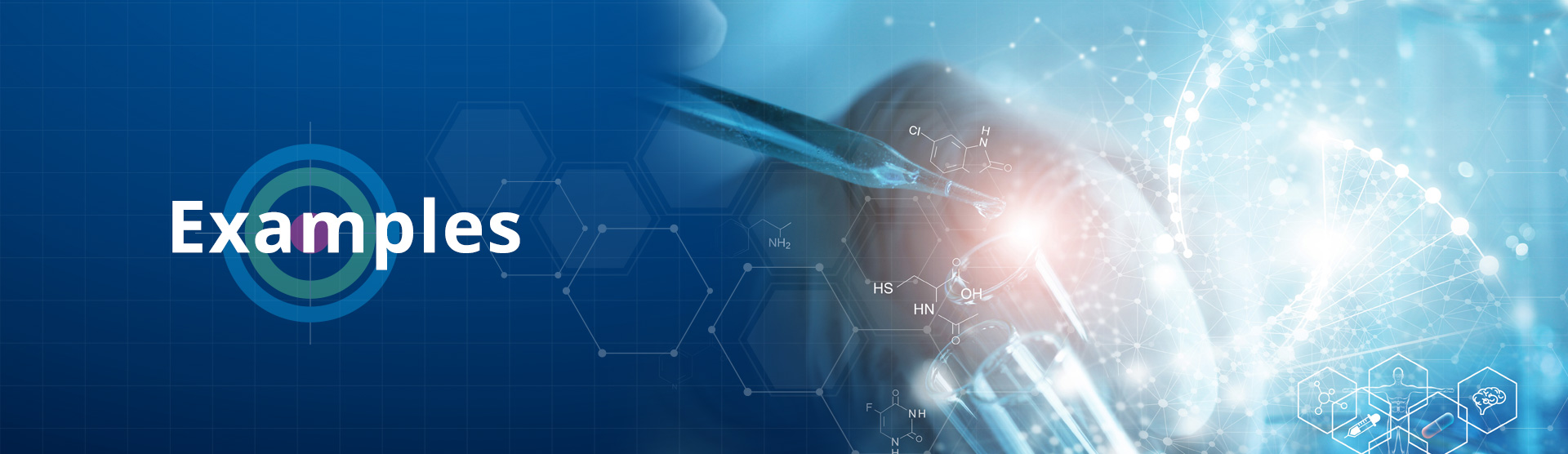
The machine learning innov’SAR platform of PEACCEL is a industry proven and efficient protein evolution tool. It has been already successfully applied in numerous collaborations (see examples) to optimise different properties of a protein. Its main advantages are :
1. It doesn’t need a extensive data set
As shown in example 1, the algorithm could develop a efficient model with only 9 single point mutations.
2. innov’SAR can predict epistatic effects of combinations of single point mutations
In example 1 and 2 we could show that innov’SAR was able to integrate the interactions between the recombined mutations correctly into its model and that it could predict the interactions and properties of new recombinants correctly.
3. The method significantly reduces time and costs of protein evolution
As the rational screening and the identification of improved variants is done in silico, only a low number (10-20) mutants have to be expressed and screened. In the cited examples only a handful of mutants were tested. The time from reception of the training set to the final testing of the improved variants can be in some cases reduced to 3 months.
4. A high number of mutants can be screened
Our current computer capacity allows to test and screen up to 1 billion mutants per day. This corresponds to the number of possible recombinations between 30 single mutations ;
5. innov’SAR can predict improved variants of proteins, even when the protein was already heavily evolved.
As can be seen in the shown examples we were able to even improve optimized proteins which already demonstrated significant improvements (15-30 fold improvement of wild-type).
Other examples can be found in PEACCEL’s scientific publications
Example 1
This example is fully described in the paper.
In this project PEACCEL improved the enantioselectivity of an epoxide hydrolase.
Challenges :
1- Limited training set with only 9 single point mutations.
2- Can innov’SAR predict epistatic effects between the point mutations?
3- Is it possible to improve the best known variants?
Result :
PEACCEL could establish a valid model with the 9 point mutations that could describe most of the epistatic effects (blind test with 28 recombinants). The model could predict mutants that were better (55x wild-type) than the best existing mutant (30x wild-type). The predictions could be successfully reproduced in the wet lab.
Example 2
In this project the PEACCEL technology could again improve an already highly optimized enzyme so that the it is now sufficiently efficient to be industrialized.
Problem :
Bifidobacterium adolescentis transglucosylase favors maltose formation to kojibiose with a selectivity of 35% for the later
– Previous work: wet lab mutation of 11 positions
– Best hit: selectivity 79%
Solution :
– « in silico » application innov’SAR to generate « super mutant »
– screen all possible combinations of wet lab tested mutations (~60 500)
– selection and testing of best hits
– Identification of new highly selective mutant: 95%
PEACCEL’s Founders Publications.